Bilevel learning in image inpainting
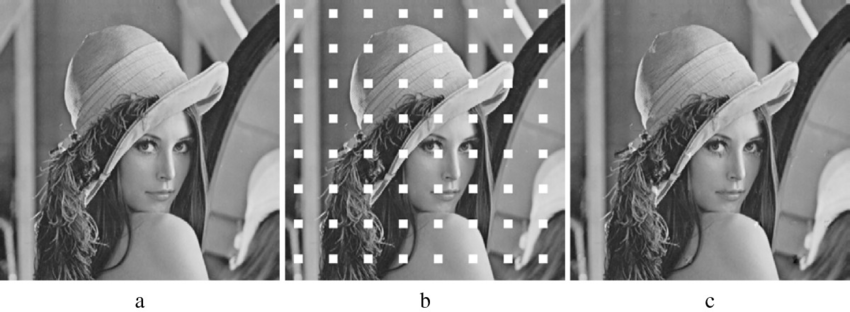
- Created by: ModeMat
- Current state: Ejecucion
- Colaboration: EPN
Junior project funded by the EPN Quito ($60.000), since January 2016.
Image inpainting is one of the most representative problems in digital image processing. This consists of restoring lost parts of an image, by mainly using information from the neighbourhood of the missing part. In the variational context this is usually acomplishe by considering local type differential operators, such as total variation, mean curvature, Euler’s elastica, among others (see [CS05] for further details).
While operators of local type, involved in variational or PDE models, are efficient in restoring small domains, they are generally not useful for filling domains that represent an important part of the image. Therefore, in recent years, the use of non-local operators for filling images has attracted increasing attention [GO08, BCM05b]. This type of operators allows to incorporate information from other parts of the image, and not only of the neighbourhood of the missing parts. Roughly speaking, missing pixels are filled with information from the beighbourhood thereof and form remote parts of the image, but with similar colour intensity patterns [BCM05b].
On the other hand, inpainting through non-local operators involves tunning of different scalar model parameters, which have a decisive effect on the final result. In recent years bilevel optimization schemes have been proposed in order to "learn" optimal parameters from a set of training images [DlRSV15, DlRS13]. Such schemes have been studied for noise filtering problems with different variational regularizers, like Total Variation, Total Generalized Variation or Infimal Convolution Total Variation.
The main goal of this project consists in stating and analyzing a bilevel optimization problem in function spaces for the optimal choice of parameters involved in image inpainting with nonlocal partial differential operators. Our study will particularly focus on problems involving operators of fractional type. The project will encompass the mathematical analysis of the problem and its numerical solution.
References
- [BCM05b] Antoni Buades, Bartomeu Coll, and Jean-Michel Morel. A review of image denoising algorithms, with a new one. Multiscale Modeling & Simulation, Vol. 4(2):490-530, 2005.
- [CS05] Tony F Chan and Jianhong Jackie Shen. Image processing and analysis: variational, PDE, wavelet, and stochastic methods. SIAM, 2005.
- [DG13] Marta D’Elia and Max Gunzburger. The fractional laplacian operator on bounded domains as a special case of the nonlocal diffusion operator. Computers & Mathematics with Applications, Vol. 66(7):1245–1260, 2013.
- [DGLZ13] Qiang Du, Max Gunzburger, RB Lehoucq, and Kun Zhou. A nonlocal vector calculus, nonlocal volume-constrained problems, and nonlocal balance laws. Mathematical Models and Methods in Applied Sciences, Vol. 23(3):493–540, 2013.
- [DlRS13] Juan Carlos De los Reyes and Carola-Bibiane Schönlieb. Image denoising: Learning the noise model via nonsmooth PDE-constrained optimization. Inverse Problems & Imaging, Vol. 7(4), 1183-1214, 2013.
- [DlRSV15] Juan Carlos De los Reyes, Carola-Bibiane Schönlieb, and Tuomo Valkonen. The structure of optimal parameters for image restoration problems. Journal of Math. Analysis and Appl., Vol. 434(1): 464–500, 2016.
- [GO08] Guy Gilboa and Stanley Osher. Nonlocal operators with applications to image processing. Multiscale Modeling & Simulation, 7(3):1005–1028, 2008.